Business Day Safety PR Safety and Security PR
Tin Ears – Machine Learning Set Against Social Engineering
Social engineering fraud (SEF) is on the rise, with the approaches more brazen and manipulative than ever before. An SEF attack refers to any transaction where a victim is tricked into either disclosing confidential financial details or transferring money to fraudsters. Common, time-tested examples of SEF include phishing, which is carried out through email, and smishing, which is perpetrated via text messaging.
With the progression of technology, criminals are forever finding new ways to commit digital banking crimes. In South Africa, data released by the SA Banking Risk Information Centre (Sabric) around June 2018 revealed that more than half (55%) of the gross losses due to crime reported were from incidents that had occurred online.
Today’s fraudsters are learning fresh tactics and acting on new platforms of which the finance industry needs to be aware.
Vishing fraud
Phone call social engineering fraud – known as vishing – has gained in popularity of late, and relies on the fraudster’s powers of persuasion in conversation with their victim.
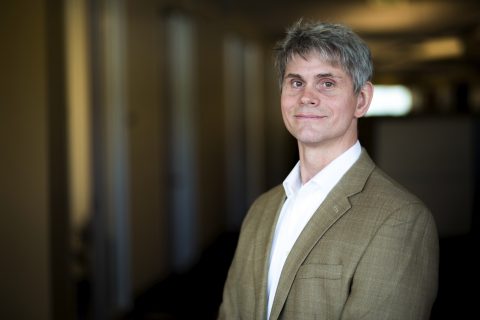
This type of SEF spikes around tax season when fraudsters claim to be the South African Revenues (SARS), and use spoofing to make the calls appear as if they originate from official phone numbers.
Victims are told that they owe tax and must pay promptly, sometimes with the threat of arrest if they do not. Alternatively, the caller may say that a refund is due and account details are needed to make payment.
Push payment fraud
Depending on how the funds are moved from a victim to a fraudster’s account, push payment fraud falls into one of two categories:
- Unauthorised push payment transactions are not authorised by the account holder. Instead, they are carried out by a fraudster using compromised account authentication details given to them by the true account holder.
- Authorised push payment scams deceive the account holder into making the payment to an account controlled by the fraudster.
This type of fraud has become more attractive to fraudsters since the advent of real-time payment schemes such as online banking transfers or cash sends. Payments made online are a little complex to revoke as they often involve the filling out of forms in order to request a reversal of funds back into your account – and with cash sends especially, the transfer happens so instantly that criminals are able to make off with the funds equally quickly.
Advancing machine learning to fight SEF
The good news is that machine learning models can counteract SEF techniques. Designed to detect the broad spectrum of fraud types attacking financial institutions today, these applications build and update behavioural profiles online and in real time.
Profiling technology
By monitoring payment characteristics such as transaction amounts and how quickly payments are being made, these models can detect both generic fraud characteristics and patterns that only appear in certain fraud types, such as SEF.
In SEF scenarios, the above-mentioned behaviours will appear out of line with normal transactional activity and generate higher fraud risk scores.
Behaviour Sorted Lists (B-LISTs)
Another advanced machine learning model feature is the Behaviour Sorted List (B-LIST). B-LISTs keep track of the way various common transactions intersect at either the customer or account level, such as:
- A list of beneficiary accounts that a customer pays regularly
- Devices that a customer has used in the past to make payments
- Foreign countries that a customer has paid in before
- A list of payers from which a customer regularly receives funds
- Typical amounts of new payment originations
- Time of day and day of week typical of payments
FICO’s research has shown that transactions made out of character are more than 40 times riskier than those that follow at least one established behaviour. B-LIST technology enables machine learning models to detect outliers based on a deep appreciation of an account-holder’s behaviour.
In the case of unauthorised push payment fraud – where the fraudster is making the payment – transactions are often made from a device not typically used by the legitimate account-holder, and the funds will likely go to a strange beneficiary account.
Following this, the fraudster might go one step further and hijack the account’s primary contact channels, locking out the true owner and taking over the entire account. Machine learning models can also track these risky non-monetary events, such as a change of email, address or phone number, which can often precede fraudulent monetary transactions.
Authorised push payments are in many ways more difficult than the unauthorised cases, and tragic. Customers can be so panicked by the social engineering fraudster that when the bank intervenes, the customer distrusts, ignores, or resists the bank’s efforts to protect the customer’s accounts.
In such situations, B-LIST technology utilises deep knowledge of typical anticipated behaviours, which is based on extensive profiling of the true customer’s past actions. We are incorporating collaborative profile technology to bring additional cross-customer understanding of the new behaviours of similar banking customers. These methods can be used to home in on individuals that are often targeted for authorised push payments and trigger the bank’s intervention.
Fraudsters have always targeted the weakest link in the banking process. As systems become more and more secure, the weakest link, increasingly, are customers themselves. However, by analysing the way each customer normally uses their account, banks can detect transactions that are out of character and stop them before any money disappears, which will make social engineering scams less profitable. Not only will customer behaviour profiling help prevent fraud in real time, but it will also enable a frictionless experience for ordinary customers, ensuring their loyalty in the long run.
Dr. Scott Zoldi is chief analytics officer at analytic software firm FICO.
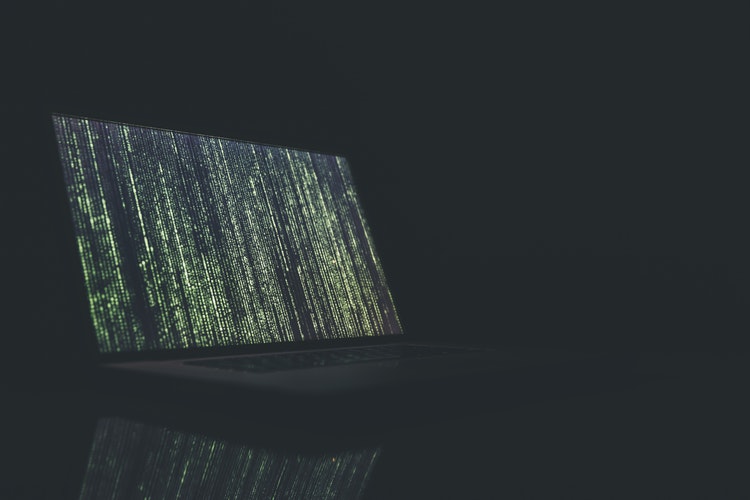