MedTech
From AI to GP
Developments in artificial intelligence (AI) have revolutionised some and touched most industries, from manufacturing and logistics to entertainment and finance. Generally, however most people vastly overestimate the ability of AI when it comes to decision-making. In medicine, breakthroughs are happening all the time. Earlier this year, tests at a Chinese clinic where doctors see 80 patients a day showed that an AI was better at picking up symptoms of childhood diseases than junior doctors.
For over a century, for example, pathologists have practised largely by staring through a microscope at images looking for anomalies. It’s time-consuming, laborious work that requires great attention to detail to identify complex patterns and aberrations that enable pathologists to diagnose diseases.
And it’s these traits that make it theoretically suitable for AI. Recently, advances in technology in the form of whole-slide imaging and data storage and transmission, as well as huge leaps forward in machine learning, have allowed practice to catch up to theory.
In 2016, a team from Harvard Medical School and the Beth Israel Deaconess Medical Centre developed methods of training AIs to analyse pathology data by building multilayered artificial neural networks. The AI achieved a 92 per cent success rate in a test involving scanning slides of lymph node cells for cancer, close to the human success rate of 96 per cent, while human and machine analysis combined yielded a success rate of 99.5 per cent.
Israeli start-up Aidoc is already using AI in this manner. Its technology analyses medical scans to help identify high-level visual abnormalities. Radiologists still examine every image, but Aidoc acts as a line of triage, helping to prioritise patients.
“We focus on time-critical conditions where a few hours or minutes can be the difference between life and death,” says Aidoc’s Ariella Shoham. “Examples include intracranial haemorrhage, pulmonary embolism and c-spine fracture.”
To date, Aidoc’s algorithms have examined imagery from more than one million patients, and been deployed in over 100 sites. Aidoc started with CT scans, but the team is adding support for oncology and X-rays.
“We’re working diligently to offer full-body coverage of acute pathologies that are time-sensitive, as well as time-consuming pathologies in which AI will help make radiologists more efficient in tedious and repetitive tasks,” says Shoham.
Where is AI needed most?
Dr Tapiwa Chiwewe, manager of the Advanced and Applied AI Team at IBM Research Africa, is working on a cancer mapping project that focuses on reducing the delay in reporting cancer statistics in South Africa. The project, which has partnered with the National Cancer Registry of South Africa, is primarily focused on breast cancer.
“Many cancer registries around the world employ expert human coders to analyse pathology reports in order to come up with an International Classification of Diseases for Oncology (ICD-O) code,” explains Chiwewe. “Because this is a manual process, there is a lag in reporting these statistics. It’s a global problem: in South Africa it takes four years, in the US it’s 1.7 years. We’re using AI and machine learning to read these pathology reports and come up with these ICD-O codes in real-time, almost instantaneously. The ultimate goal is to use this data to inform public health planning and intervention programmes. How can government officials make policy when they’re dealing with data that is four years old?”
Based on the prevalence of cancer, perhaps the relevant authorities can decide if they need to invest more in training certain types of oncologists, or building certain treatment facilities. “Having better situational awareness of cancer helps with resource and intervention planning,” says Chiwewe. “Also, imagine there’s a spike in a particular type of cancer in a particular part of the country, that raises questions. With a four-year delay, it’s too late to investigate that.”
Helping physicians and patients
IBM has come in for a lot of criticism over the last year because its much-vaunted Watson has failed to match initial expectations about its potential to revolutionise healthcare. But Dr Mehool Patel, associate chief medical officeer at IBM Watson Health Oncology and Genomics says that the platform is showing value.
Patel stresses that one of the primary goals of IBM Watson is to be a clinical decision support tool. “It’s never meant to replace the physician. But its recommendations can help doctors support their decisions and give them further leverage to reassure patients that they are receiving the best possible treatment.”
More medical research is being conducted and more data generated than ever before. That’s a good thing. But it’s also creating a tidal wave of information that is difficult to corral and process. A study by the International Data Corporation estimated that medical data will reach a mind-boggling 2.3 trillion gigabytes by 2020.
“Our literature data evidence team has created a structure to scan through databases and formulate a hierarchy with the most relevant papers at the top,” explains Patel.
Millions more articles and datasets are published every year, in every medical field, and AI is going to have a role in helping doctors to navigate information overload.
For diagnostics, things are developing all the time, but it’s clear that there’s a long way to go.
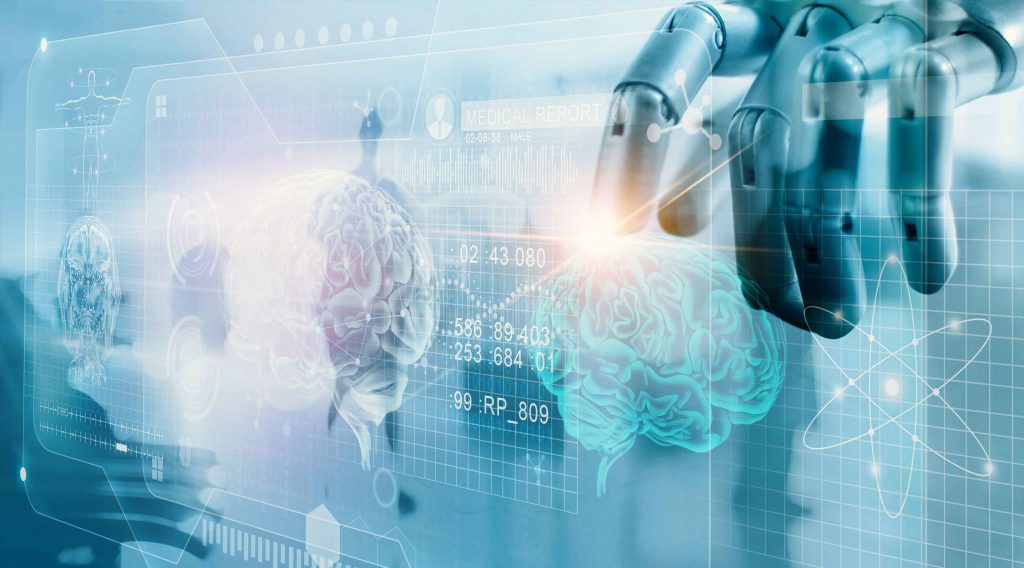